If you search for Data-driven lead scoring for b2b on Google, you'll get over 8,480,000 results. I think it's fair to say that there is enough content about this to drive most people mad. You'll find results ranging from websites claiming that you can set up a Data-driven lead scoring model in 20 minutes to websites trying to sell you AI-driven lead scoring models that look great on paper but break as soon as you hit the publish button.
To find out which is suitable for you, you need to apply first-principle thinking and deconstruct Lead Scoring.
What is the goal of lead scoring?
Let's start with defining Lead Scoring. According to Wikipedia, Lead scoring is a methodology used to rank prospects against a scale that represents the perceived value each lead represents to the organization. Basically, you assign scores to your contacts based on different attributes.
What should be included in a good lead scoring model?
There are a few different ways to look at Lead Scoring.
Lead Scoring grouping criteria
Explicit scores - Information that the Contact provides you. E.g. When they fill out the Job Title field on a web form.
Implicit scores: Behaviour you track to determine intent. E.g. a visit to the pricing page or increased activity on the website.
A helpful way to think about these is that the Explicit scores can give you information about the Prospect Fit, (meaning: the alignment between your product and a Contact’s needs and/or is the Contact part of your Ideal Customer Profile?) whereas the Implicit scores help you understand intent or interest.
You can also capture a wealth of Explicit information using third-party tools such as ZoomInfo to enrich the data. We use it with a few clients to reduce the number of fields required in forms and increase the conversion rate.
We further break down the criteria as follows:
- Contact behavior
- Contact demographics
- Company information
The most notable thing here is that there's been a consistent (and rightful) transition to an Account-Based approach in all the B2B activities in recent years. That means that we try to assign more value where we can identify that multiple contacts from the same organization show an interest in the product. Almost like Bombora does when calculating their Surge score.
Examples of lead scoring attributes
Contact behaviour lead scoring attributes examples:
- Webpage visit
- Blog post page
- Blog subscription
- Case Study page view
- Webinar registration
- Webinar attendance
- Product datasheets
- Webchat engagement
- Community participation (e.g. submitted of voted on an idea)
- Social Media engagement
For each of the above, you can score different engagement levels:
- Viewed
- Viewed multiple times
- Downloaded
- Downloaded multiple times
- Clicked link
- Scheduled demo
- Attended demo
- etc
Contact demographic lead scoring attributes examples:
- Title
- Role
- Type of email used (free or business email)
- Department
- etc
Company information lead scoring attributes examples:
- Industry
- Number of employees
- Company revenue
- Revenue growth
- Location
- Financial Year end
- Technology used
- Current solution they have in place
This can quickly get out of hand, so analyzing the data before implementing hundreds of rules is important. Remember that you will need to maintain whatever Lead Scoring Model you implement. We help our customers maintain their Lead Scoring models, but you might not have a team like ours at hand (yet).
Types of Lead Scoring
There are two types of Lead Scoring solutions on the market:
- Predictive lead scoring
- Rules-based lead scoring
They each have their pros and cons and one isn’t necessarily better than the other.
Should you use predictive lead scoring?
In case you made it this far without knowing what Predictive Lead Scoring is, Microsoft defines Predictive Lead Scoring as a predictive machine learning model to calculate a score for all open leads.
A good lead scoring model should give you a list of Lookalikes of your existing customers; Contacts who share similar characteristics to your Customers. To do this, you absolutely have to use predictive models. You also have to start from a large enough dataset of existing customers. If you're in a B2B niche and work with small volumes of clients, you can look at Opportunities instead.
However, you mustn't trust the data blindly.
When we propose Data-Driven Lead Scoring models, we see some resistance from people that think we'll simply throw in the data into a Business Intelligence tool, process it and then implement the scores generated without further analysis. That would be disastrous. While big data is fantastic, it has one big flaw: it only tells you what happened in the past. For example, in 2009, Nokia said: "We have millions of data points, and we don't see any indicators of anyone wanting to buy a smartphone", and dismissed the growth of the iPhone.
Here's an interesting video about the power of not blindly trusting big data:
So big data is helpful, but you want to consider the business's changes. Have you released a new product? Have you pivoted at all? Are you looking to expand into new territory?
If the answer to any of these questions is yes, you might want to trust your team's anecdotes and opinions more than the data. If your business and its goals are static, you might want to trust the data more.
Should you use rule-based lead scoring?
Rule-based lead scoring is where it all started. A traditional Lead Scoring model uses simple rules based on desired or undesired characteristics of the Contacts.
They are easy to build and implement, depending on the platform you use and fast. We recommend them to companies that are just getting started with Lead Scoring or to those that don't have enough data to make an informed decision.
Why not both?
What we actually end up using most of the time is a mix of the approaches described above.
While the model we usually implement is not pure Predictive Lead Scoring, it is closer to it than it is to Rule-Based Lead Scoring.
With few exceptions, we stay away from implementing rules that look at a single attribute because it’s usually a bad idea to only look at one attribute or one metric because you can over-optimise for one attribute or metric and lose track of the bigger picture; this is an interesting article about pairing metrics in analytics and I believe more business areas can benefit from implementing a similar approach. But I digress… So we group attributes and then score them. Individually, some of the attributes we score might be irrelevant or might not be an indicator of success. When paired with other attributes, however, they unlock a lot more meaning.
Here’s what a typical implementation of our Lead Scoring looks like:
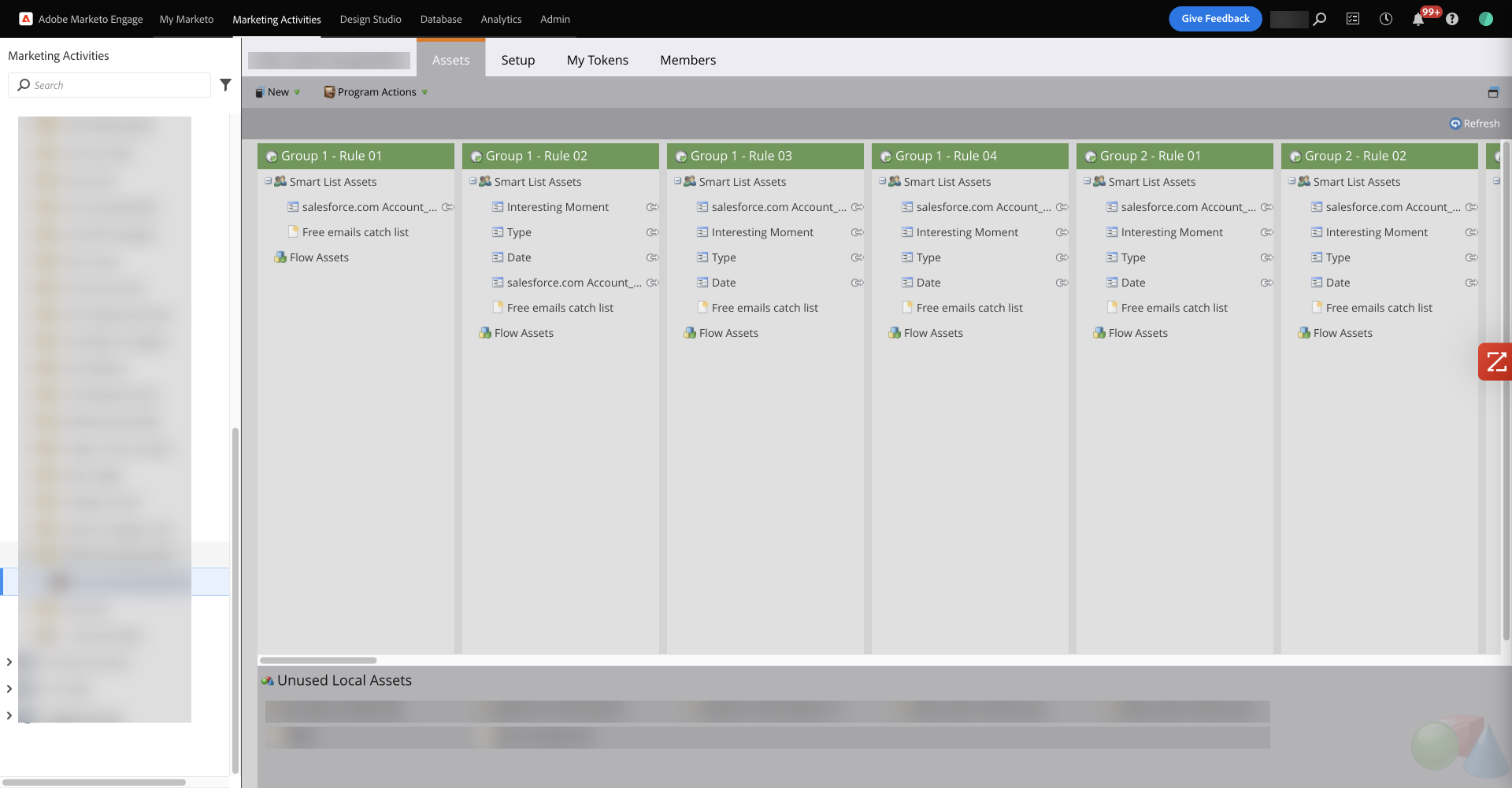
Then, you simply map the Marketo form to the Salesforce field:
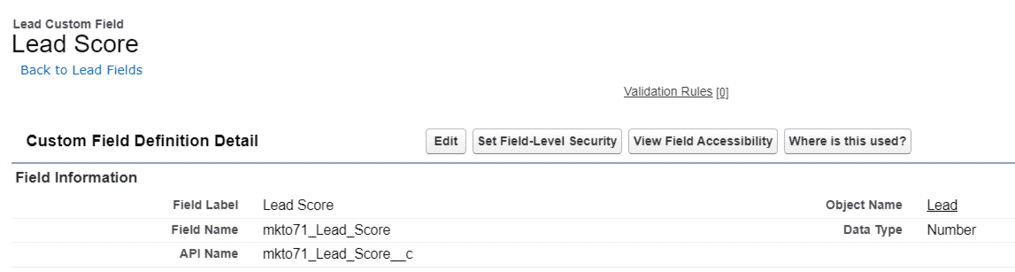
Is lead scoring worth it?
The short answer is yes, absolutely.
We wouldn't be writing this post if we thought it wasn't. We believe that B2B lead scoring is an absolute necessity in this day and age. If you haven't got a model in place, you should get one yesterday. If you have one, you should be asking yourself if it works well or if your circumstances changed. Using an old Lead Scoring model might mean you're leaving money on the table.
Not using Lead Scoring at all means you are missing out on valuable data and you’re hurting your bottom line. By not knowing which leads are valuable to you, it means you cannot optimize your Lead Generation processes to focus on the right leads and you risk wasting your sales team’s valuable time.
What do I need to get a great Lead Scoring model?
You need some data. As much data as you can gather about your Ideal Customer Profile, the behaviour of your prospects, and information from your Sales teams.
When building a Leads Scoring model, you want to see the similarities between your customers so you can create a lookalike model. But you also want to see the similarities between the prospects that never become customers. The survivorship bias example from Abraham Wald is a fitting example here because you want to see where there are no bullet holes:
Before you get started, you'll want to ask yourself who else needs to buy into the Lead Scoring model. The typical stakeholders are:
- CRM Owner
- Marketing Owner
- Sales Owner
What are my options?
There are plenty of options when it comes to B2B lead scoring
A few companies offer Lead Scoring As a Service. And, of course, HubSpot Marketo and Salesforce and pretty much any respectable Marketing Automation or CRM tool provide some form of Lead Scoring capabilities.
There are also a few Sales Enablement SaaS providers that offer Lead Scoring too.
But the choice can be overwhelming.
There's always the eternal "Build vs Buy" dilemma. While a SaaS tool can bring speed to your deployment, is that speed worth anything if the model isn't as good as it should be?
Over the years, we learned that the best Lead Scoring Model is one that is personalized to the company. Off-the-shelf solutions made grand promises and delivered little, so we usually build custom models for our clients.
We're biased, but an obvious choice would be to speak to us and see if we can build and implement your Lead Scoring Model.
Why Macro? Can't I do it myself?
We're not going to sit here and claim that this is not something you can't do yourself or together with your team. You made it so far into this post and that tells me you're both capable and interested enough to do it. But you probably shouldn't.
To use an analogy, if I do enough research, I'm sure I can change the brake pads on my car. But it's cheaper and safer to let a mechanic do it. This might be a bit extreme, but you get the idea. We've done this a few times for a few clients in different industries. Our team has skills that might not be native to your team, and because we're optimized to deliver this service, it will almost definitely be quicker.